In an era driven by technological advancements, artificial intelligence has emerged as a transformative force, reshaping industries and redefining the way we work. Among its various branches, generative AI stands out remarkablly, and poised to disrupt the workplace in unprecedented ways.
With its ability to generate human-like content, such as text, images, and videos, generative AI has the potential to revolutionize productivity, enhance creativity, and reshape the dynamics of the modern workplace.
According to Goldman Sachs,
Shifts in workflows triggered by these advances could expose the equivalent of 300 million full-time jobs to automation
It continues,
roughly two-thirds of U.S. occupations are exposed to some degree of automation by AI
In this article, we will delve into the many ways generative AI is poised to disrupt education, and transform the way we work. We will also discover how it will pave the way for a future where human and machine collaboration takes center stage.
Exactly What is Generative AI?
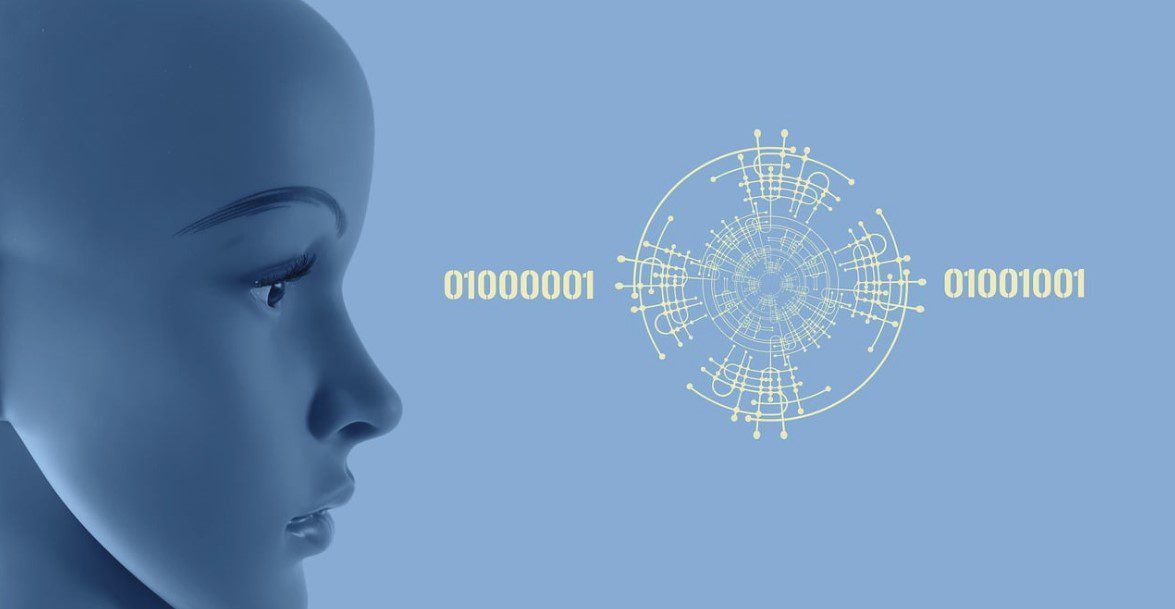
Generative AI refers to a subset of artificial intelligence that focuses on creating new and original content. Unlike traditional AI systems that are designed to recognize patterns and make decisions based on existing data, generative AI aims to ‘generate new data or content that resembles what a human would create’.
Generative AI algorithms are trained on large datasets to learn patterns and structures present in the data.
They then use this knowledge to generate new text, images, videos, or even music. These algorithms can produce content that is highly creative and often indistinguishable from what a human might produce.
One popular approach in generative AI is the use of generative adversarial networks (GANs). GANs consist of two neural networks: a generator network and a discriminator network.
A generative adversarial network (GAN) is a machine learning (ML) model in which two neural networks compete with each other by using deep learning methods to become more accurate in their predictions.
The generator network creates new content, while the discriminator network evaluates the generated content and tries to distinguish it from real human-generated content.
Through an iterative training process, the generator network learns to produce content that becomes increasingly difficult for the discriminator network to differentiate.
Generative AI has been used in various applications, including natural language generation, image synthesis, video production, and more.
Here are the most popular branches of Generative AI to date:
Generative AI encompasses several branches that focus on different aspects of generating content:
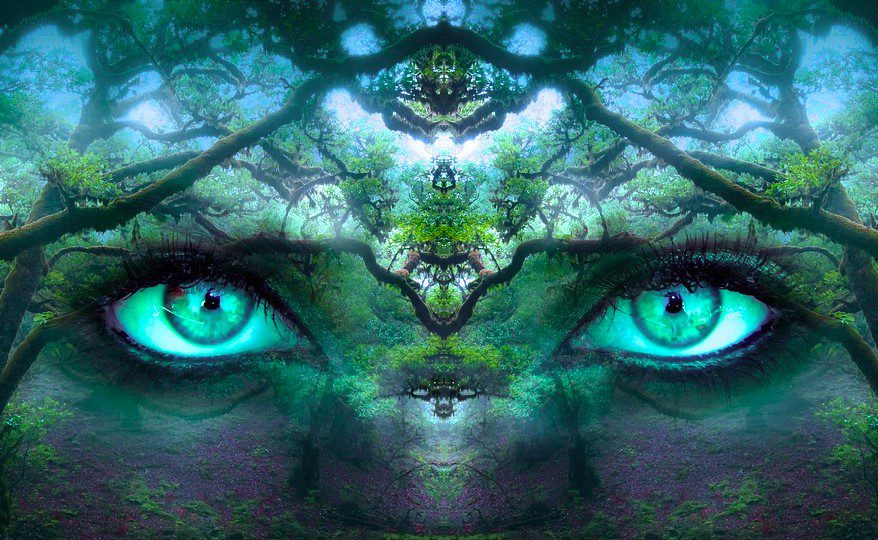
Image Generation:
This branch focuses on generating new images using generative AI techniques. It includes subfields like image synthesis, image completion, and image manipulation. Techniques such as GANs, VAEs, PixelCNN, and flow-based models are commonly used for image generation tasks.
A GAN model is trained on a dataset of human faces. The generator network learns to generate synthetic faces, while the discriminator network tries to differentiate between real and fake faces.
Through iterative training, the GAN model improves its ability to generate realistic human faces.
Text Generation:
Text generation involves generating coherent and contextually relevant text. This branch includes tasks like language modeling, machine translation, text summarization, dialogue generation, and story generation.
Autoregressive models, Seq2Seq models, and transformers are often employed for text generation tasks.
GPT (Generative Pre-trained Transformer) Models: GPT models, such as GPT-3, are state-of-the-art language models that employ the Transformer architecture. GPT-3 is a powerful generative AI tool that can generate coherent and contextually relevant text across various domains. It has been used for tasks like content creation, chatbots, and creative writing.
For example, GPT-3 can generate a news article based on a given prompt or continue a story with imaginative and engaging text.
Music Generation:
Music generation is a branch of generative AI that focuses on creating new musical compositions or generating music in a specific style. Recurrent neural networks (RNNs), variational models, and generative models trained on musical data are commonly used in this area.
Magenta’s MusicVAE is a generative AI tool that can be used to generate musical melodies. It uses a Variational Autoencoder (VAE) architecture to learn a latent space representation of musical sequences and can generate new melodies based on that learned representation.
Video Generation:
Video generation involves creating new video content or generating videos that follow a certain style or pattern. It often requires models capable of understanding temporal dependencies and generating coherent sequences of frames.
GANs and recurrent neural networks are commonly used for video generation tasks.
DeepArt, an AI tool based on neural style transfer, can be used to apply artistic styles to existing videos, creating visually appealing and unique effects.
Another example of a generative AI tool used to generate videos is DeepMind’s “DALL-E” model. While DALL-E is primarily known for generating images, it can also be extended to generate short video sequences by combining its image generation capabilities with techniques like frame interpolation.
Speech Synthesis:
Speech synthesis involves generating human-like speech or converting text into spoken words. This branch includes technologies like text-to-speech (TTS) synthesis and voice cloning. Deep learning models, including autoregressive models and neural vocoders, are employed for speech synthesis tasks.
One popular generative AI tool used for speech synthesis is Tacotron 2. Tacotron 2 is an advanced neural network-based model that can generate human-like speech from given text input.
Simulation and Virtual Environments:
Generative AI is also used to create simulated environments or virtual worlds. These environments can be used for training AI agents, testing scenarios, or providing immersive experiences.
GANs, reinforcement learning, and physics-based models are often utilized in this branch.
One popular generative AI tool used for generating simulations and virtual environments is Unity ML-Agents. Unity ML-Agents is an open-source toolkit developed by Unity Technologies that combines
Unity, a popular game engine, with machine learning algorithms to create intelligent agents and immersive virtual environments.
Creative Applications:
Generative AI has gained significant attention in creative fields, including art, design, and fashion. It involves using AI algorithms to generate artwork, design elements, fashion designs, and other creative outputs. GANs, style transfer, and other generative models are employed for creative applications.
These branches of generative AI illustrate the diverse range of applications and domains where generative models are utilized, and where they will disrupt productivity.
Each branch has its unique challenges and techniques, contributing to the advancement of generative AI as a whole.
Sectors Where Generative AI Will Disrupt the Workplace!
Generative AI, with its ability to generate human-like text, images, and even videos, has the potential to significantly disrupt the workplace across various industries.
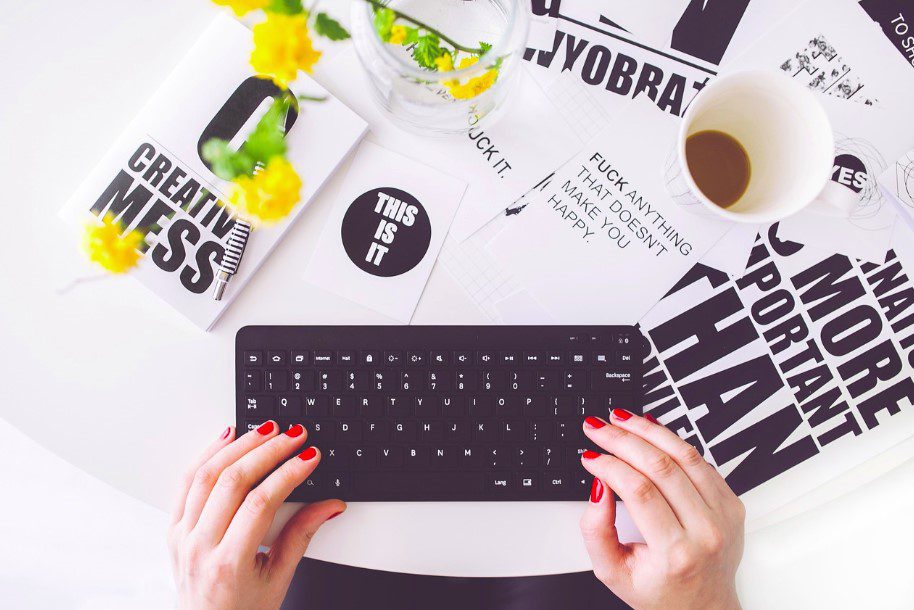
Here are some ways in which the new tech is poised to transform how we work and live:
1. Content Creation:
Generative AI can automate the creation of written content, such as articles, reports, and social media posts. It can generate high-quality text that closely resembles human-written content, saving time and effort for content creators.
Using tools such as OpenAI’s ChatGPT, users can create content that is similar to what humans can.
However, this may also raise concerns about authenticity, plagiarism, and the potential for misuse.
2. Design and Creativity:
AI-powered design tools can assist in generating creative content, ranging from logos and graphics to website layouts. By leveraging generative AI algorithms, designers can receive suggestions, inspiration, or even fully generated designs that align with their specifications. This can streamline the design process and boost creativity.
3. Customer Service:
Chatbots and virtual assistants powered by generative AI can handle customer inquiries and provide support around the clock. They can understand and respond to user queries in natural language, offering personalized assistance and freeing up human agents to focus on more complex tasks. This automation can enhance customer service efficiency and scalability.
4. Data Analysis:
Generative AI algorithms can analyze vast amounts of data and generate meaningful insights. They can identify patterns, make predictions, and offer data-driven recommendations. This can expedite decision-making processes, optimize operations, and provide organizations with a competitive advantage.
5. Virtual Collaboration:
Generative AI can facilitate virtual collaboration by automating certain tasks in remote teams. For instance, AI can summarize meetings, generate action items, and provide real-time language translation, allowing team members to communicate and work more effectively across language barriers and time zones.
6. Personalization and Recommendations:
By analyzing user behavior and preferences, generative AI can provide highly personalized recommendations for products, services, and content. This can enhance user experiences, drive customer engagement, and boost sales by tailoring offerings to individual needs and preferences.
7. Training and Education:
AI-powered tools can generate interactive learning materials, simulations, and virtual tutors. These tools can adapt to individual learners’ needs, provide immediate feedback, and offer personalized learning paths.
Generative AI can democratize access to education and training, making learning more engaging and accessible.
The Implications of Generative AI in the Workplace
The disruptive nature of generative AI on the human workforce are multifaceted and can have both positive and negative effects.
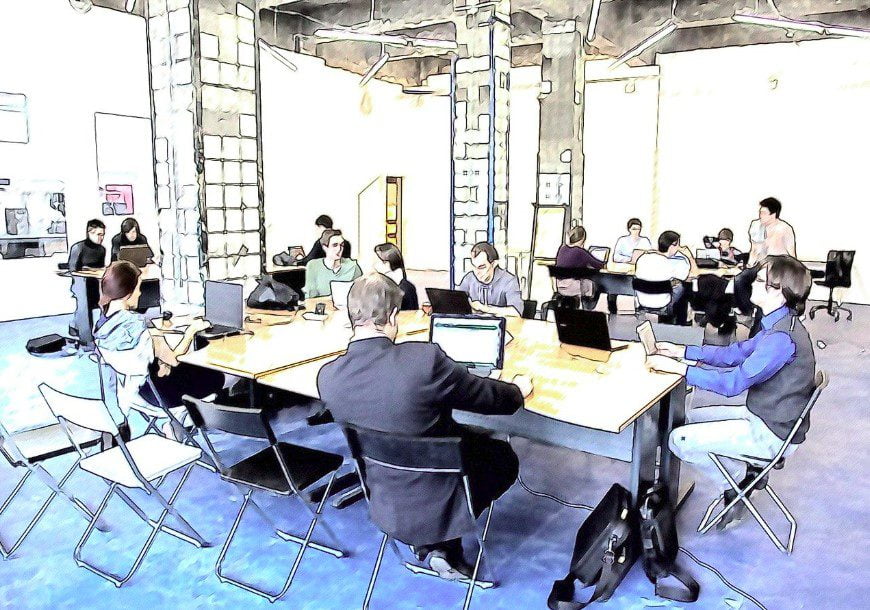
Here are some key points to consider:
1. Automation of Repetitive Tasks:
Generative AI has the potential to automate repetitive and mundane tasks that don’t require human creativity or decision-making. This can free up human workers to focus on more complex and strategic work, leading to increased productivity and efficiency.
2. Job Displacement:
As generative AI continues to advance, it will disrupt certain jobs that primarily involve routine tasks may become obsolete. Workers in industries like manufacturing, data entry, or customer service might face displacement as AI systems can perform these tasks more efficiently.
However, new job opportunities may also emerge as AI technology creates new industries and demands different skill sets.
3. Skill Shift and Reskilling:
The integration of generative AI into the workforce will likely require workers to develop new skills to adapt to changing job requirements. Jobs that involve human-AI collaboration, such as AI system oversight, data analysis, or algorithm design, may become more prevalent.
To stay relevant, individuals may need to reskill or upskill to fulfill these new roles.
4. Creative Augmentation:
Generative AI can also enhance human creativity by assisting in the generation of content, design, or artwork. For instance, AI-based tools can help artists, writers, and designers with ideas, suggestions, or initial drafts, enabling them to be more productive and explore new creative possibilities.
5. Ethical Considerations:
The development and deployment of generative AI raise ethical concerns. Ensuring the responsible and ethical use of AI systems becomes crucial, including considerations of bias, privacy, and transparency.
Human oversight and regulation will be essential to mitigate any negative impacts and protect against misuse.
6. Economic Disparity:
The adoption of generative AI could exacerbate economic disparities. Individuals with access to AI technology and the ability to adapt to its integration in the workforce may benefit significantly.
However, those lacking access or the necessary skills may face challenges and widening inequalities.
Overall, the implications of generative AI on the human workforce are complex and require careful attention to ensure a smooth transition, mitigate negative impacts, and harness the technology’s potential for the benefit of society as a whole.
The Way Forward for Humans!
When different kinds of generative AI tools disrupt human employment, it is important to consider several strategies moving forward.
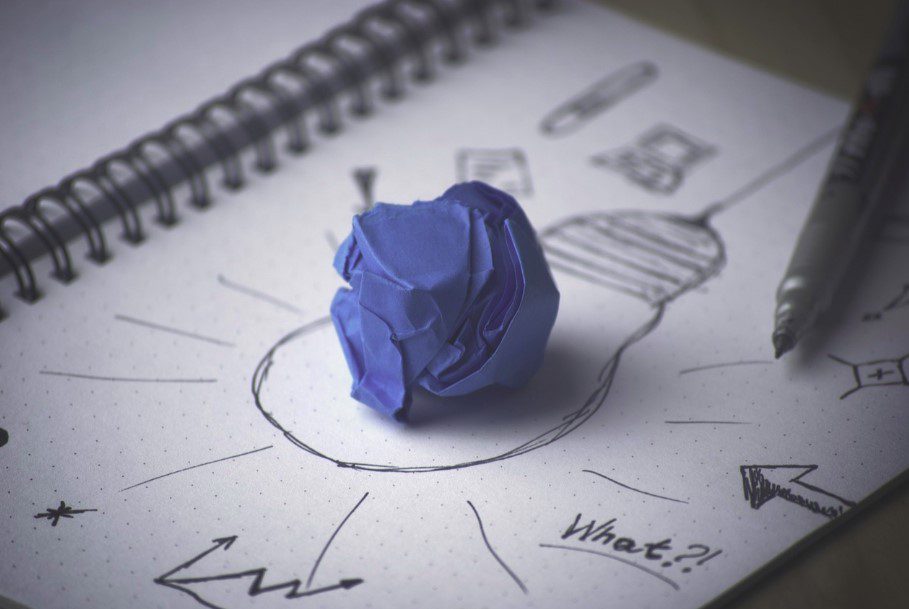
Here are some key aspects to focus on:
1. Education and Skill Development:
Investing in education and skill development is crucial. Preparing the workforce for the changing job landscape involves equipping individuals with the necessary skills to collaborate with generative AI systems effectively.
This may include training in 21st century literacies such as AI literacy, data analysis, algorithm design, and human-AI interaction.
2. Reskilling and Upskilling Programs:
Implementing reskilling and upskilling programs can help displaced workers transition into new roles. Governments, educational institutions, and companies should collaborate to provide training initiatives and resources to help individuals acquire the skills needed for emerging job opportunities.
3. Entrepreneurship and Innovation:
Encouraging entrepreneurship and fostering innovation can help create new job opportunities and industries. Supporting startups and providing resources for individuals to start their own businesses can mitigate the impact of job displacement and promote economic growth.
4. Social Safety Nets:
Establishing robust social safety nets is crucial to support individuals affected by job disruption. This may include unemployment benefits, income support programs, healthcare provisions, and other forms of assistance to ensure a smooth transition for displaced workers.
5. Ethical Frameworks and Regulations:
Developing and enforcing ethical frameworks and regulations around the use of generative AI can protect workers’ rights, ensure fairness, and prevent misuse of the technology.
Governments, policymakers, and industry stakeholders need to collaborate to establish guidelines and regulations that address potential risks and protect the interests of both workers and society.
6. Human-AI Collaboration:
Emphasizing the importance of human-AI collaboration rather than complete replacement is vital. Recognizing the unique strengths of both humans and AI systems and finding ways to integrate them can lead to more productive and meaningful work experiences.
This involves designing AI systems that augment human capabilities, encourage creativity, and support decision-making processes.
7. Continuous Adaptation and Lifelong Learning:
The nature of work is evolving rapidly, and individuals must embrace continuous adaptation and lifelong learning. Encouraging a culture of continuous learning and providing accessible avenues for individuals to update their skills will be essential to thrive in an AI-disrupted job market.
By focusing on the above strategies, we can navigate the challenges posed by generative AI and work towards creating a future where human workers and AI systems can coexist harmoniously.
This will leverage the unique strengths of each to drive innovation and progress.
The good news is
jobs displaced by automation have historically been offset by the creation of new jobs,
What Next for Humans?
As generative AI becomes more sophisticated, it continues to raise ethical concerns that need answers.
There are challenges related to the authenticity and ownership of generated content, potential biases in training data, and the responsible use of AI-generated content.
These considerations require careful attention to ensure that generative AI technologies are developed and deployed in an ethical and responsible manner.
Alfred Amuno
Source link